For decades, colleges, government agencies and foundations have experimented with recruitment and retention efforts designed to increase diversity in engineering programs.
However, the efforts have not significantly boosted the number of women, students of color, individuals with disabilities and other underrepresented groups studying and earning degrees in STEM and engineering fields.
Latino, Black, Native American and Alaska Native students are underrepresented among science and engineering degree recipients at the bachelor’s degree level and above. The groups are also underrepresented among STEM workers with at least a bachelor’s degree.
Women are also underrepresented in the STEM workforce and among degree recipients in engineering and computer and information sciences.
I study equity and social justice in STEM learning. In my recent study, I found that more students from diverse backgrounds could excel in engineering programs if course content were tailored to a wider variety of learning preferences.
Why it matters
Morsa Images/Getty Images
During my time as a program officer at the National Science Foundation, an independent federal agency that supports science and engineering, I reviewed plenty of research focused on broadening participation and diversifying student enrollment in STEM fields.
Progress can stall on efforts to boost diversity because college instructors do not consider the synergistic relationship between the content and the learner.
Teachers are the mediators, and it is students’ experiences with the curriculum that matter.
It was long a common belief that students have different learning styles. These included kinesthetic, learning through hands-on experiences and physical activity; auditory, learning by listening to information; and visual, learning by seeing information.
More recent research does not support the idea that teaching students according to their learning style leads to improved learning.
That’s why I prefer the term “learning preferences” rather than learning styles. We all have preferences – whether for ice cream flavors, home decor or how we receive information, including how we learn.
Learning preferences are broader and more flexible, allowing multiple ways of engaging with content.
For example, let’s say a teacher always presented equations in a classroom and the student just could not get it. However, it was the only way the information was presented. To the individual learner, they have failed. Some people would say, “These kids can’t learn,” and subsequently counsel the student out of the class.
Then, years are spent repeating the same cycle.
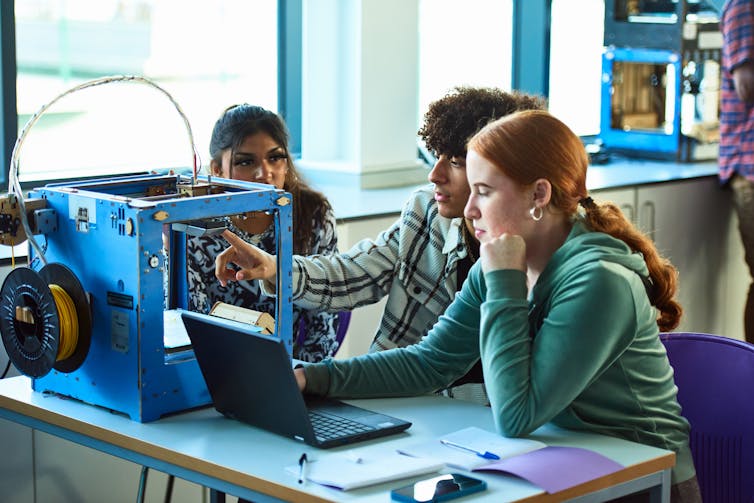
10’000 hours/Getty Images
However, educators can broaden their viewpoints if they look at the students as customers. If a customer is shopping for a shirt, they look for one that catches their eye. Ultimately, they find one they like.
Instructors need to take the same approach when trying to help students understand what is happening in class. For instance, if I have trouble with equations, I should be provided with options to engage with the lesson in ways that align with my learning preferences.
What’s next?
Learning styles have been heavily researched. However, content preferences have not been well explored.
In a truly democratic education system, curriculum design should reflect the voices of all stakeholders and not just those in positions of power, namely instructors.
Using data mining and artificial intelligence, educators have a variety of options for creating content for the various preferences a learner may want or need. For example, if a student prefers other representational content, such as word problems, graphics or simulations, AI can create diverse representations so that the learner is exposed to a variety of representations.
I argue that future studies need to consider the use of technologies such as adaptive learning applications to understand students’ learning preferences.
Prioritizing diverse learning perspectives in STEM could help create a more inclusive and responsive learning environment.
The Research Brief is a short take on interesting academic work.